FACT: A Scalable Cyber Ecosystem for Acquisition, Curation, and Analysis of multispectral UAV image data
A current cyber-agriculture data need is to improve and standardize data collection protocols and to develop curation processes and infrastructure to support the ability of machine learning (ML) to contribute to disease detection and mitigation in crops. This project will design and deploy a scalable, sustainable, data infrastructure platform that supports the data acquisition, curation, and hosting of data (primarily spectral images) collected from small unmanned aerial systems (sUAS) and will apply big data analytics modeling to this collection using deep learning (DL) to identify diseases in crops. Project objectives include:
(1) Develop a standardized approach for sUAS-based multispectral data collection,
(2) Develop a scalable cyberinfrastructure system for data curation, and
(3) Develop and deploy cloud based, DL algorithms for disease detection.
The project & goals and outcomes will develop a publicly available and curated digital ecosystem of labeled plant stress data, that is accessible through cloud-native computing and empowers users nationwide to accurately and rapidly identify and quantify plant diseases in multiple crops (through transfer learning), and further build the broader community of shared resources. This project will enable a systems approach to disease identification and empower various communities (research, farmer, industry) to effectively utilize and manage data for informed data-based decision making. The broader outcomes of this work will enable production of germplasm that are more resistant to critical biotic and abiotic stresses allowing sustainable farming. Furthermore, the information and communication technology (ICT) tools that are developed will simultaneously enable precision farming, improve profitability, and increase sustainability.
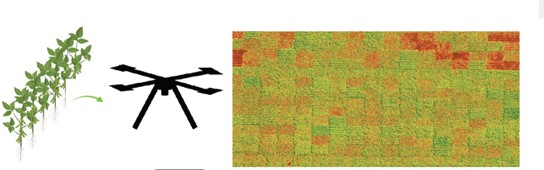